Zhen Fang (Lecturer at UTS-AAII)
Home
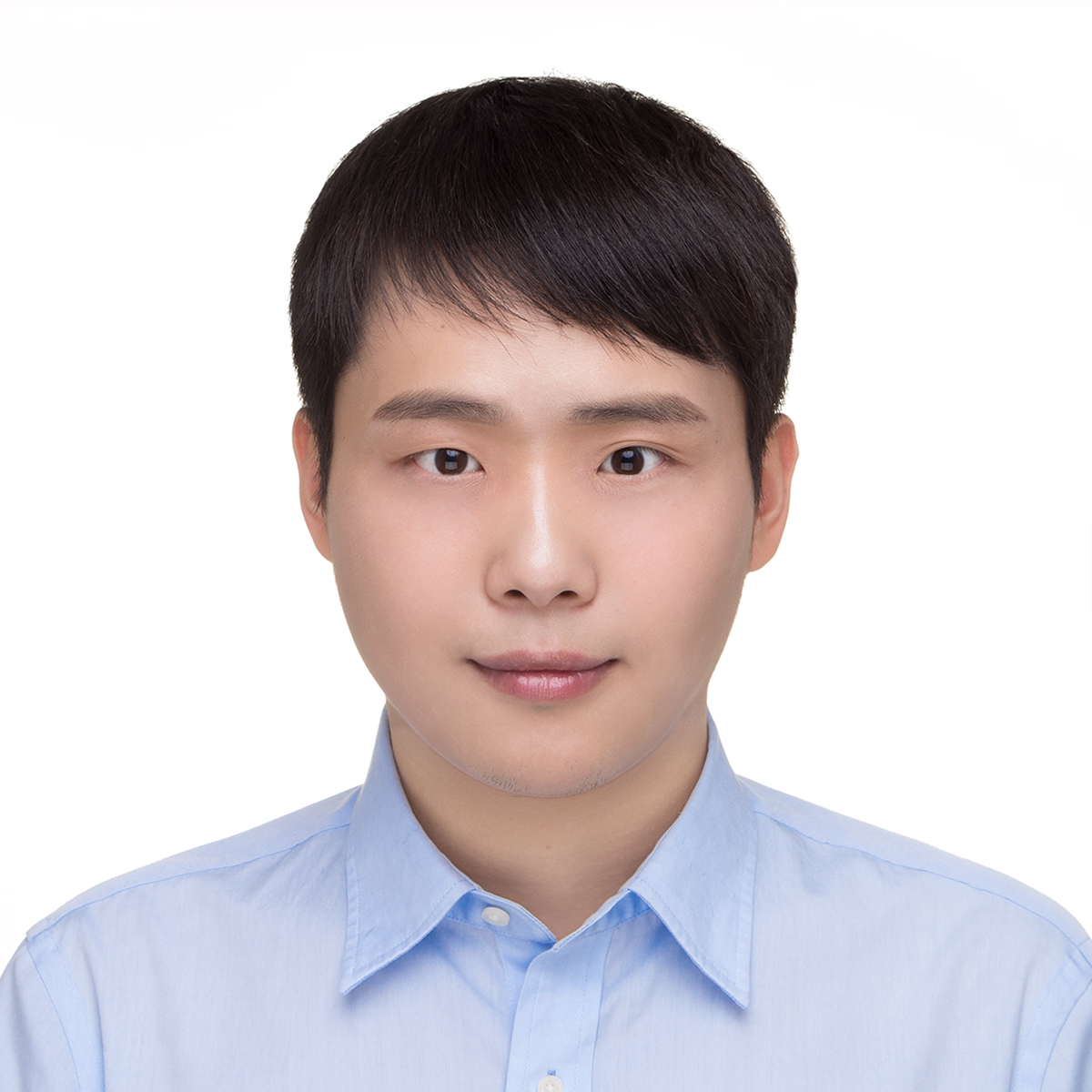 |
Zhen Fang
Lecturer (Assistant Professor) @ DeSI Lab,
ARC Discovery Early Career Researcher Awardee,
Australian Artificial Intelligence Institute,
University of Technology Sydney, Australia
Address: Level 12, UTS Central,
61 Broadway, Ultimo, Sydney, NSW2007, Australia.
E-mail: zhen.fang [at] uts.edu.au
[Google Scholar]
[CV]
I am always looking for highly self-motivated RA/Research Master/PhD students. Thanks!
Drop me an email if you are interested in my RA/Research Master/PhD. Please use the subject format [Name_Position_AppliedUniversity]. For example, [Jack_PhD_UoM], [Mike_PhD_HKU].
If we match, I will provide a full scholarship(AU$152,000 (after tax))to support your PhD study.
|
Biography
I earned my PhD from the Faculty of Engineering and Information Technology at the University of Technology Sydney (UTS), Australia. I am a member of the Decision Systems and e-Service Intelligence (DeSI) Research Laboratory at the Australian Artificial Intelligence Institute, UTS. Currently, I serve as a lecturer at UTS. My research interests include transfer learning and out-of-distribution learning. I have published numerous papers in top-tier conferences and journals, including NeurIPS, ICML, ICLR, IEEE TPAMI, and JMLR, focusing on out-of-distribution learning and transfer learning. My first-author paper received the NeurIPS 2022 Outstanding Paper Award, and I have also been honored with the ARC Discovery Early Career Researcher Award.
Research Interests
My research interests lie in Machine Learning, Transfer Learning, Statistical Learning Theory, Generalized Out-of-Distribution Learning, Foundation Models. Specifically, my current research work center around four major topics:
Transfer Learning: Transferring knowledge from a source domain to a target domain.
Statistical Learning Theory: Estimating the generalization error of a given problem or algorithm.
Generalized Out-of-Distribution Learning: Learning a generalized well model or learning a model with OOD detection abaility.
Foundation Models: Exploring the basic theory and algorithms for foundation models.
Research Experience
Lecturer (Research Intensive) (02.2023--11.2023)
Advisor: Dist. Prof. Jie Lu
Project: Transfer Learning
Postdoc (07.2021--02.2023)
Advisor: Dist. Prof. Jie Lu
Project: Transfer Learning
Research Assistant (06.2021--07.2021)
Advisor: Dist. Prof. Jie Lu
Project: Transfer Learning
Sponsors
|